New research suggests the extinction crisis may be even worse than we thought. More than half of species that have so far evaded any official conservation assessment Study are threatened with extinction, according to predictions by researchers from the Norwegian University of Science and Technology.
Conservation resources are limited and it is not feasible or logical to protect every square kilometre of land and sea. So to mitigate the rapid loss of biodiversity, where should our conservation resources go? To answer this question we first need to know which species to protect.
The International Union for Conservation of Nature Study coordinates a network of scientists. Who have assessed biological information available for all sorts of species worldwide for more than 50 years. Publishing their findings in the Red List of Threatened Species. Its goal has been to identify species that need protection with an assigned conservation category of extinction risk.
Red List that confirmed
It’s the Red List that confirmed tigers are officially endangered, for instance, or that giant panda populations have recovered enough to move from endangered to merely vulnerable.
However, while species like pandas and tigers are well studied, researchers don’t know enough about some species to properly assess their conservation status. These “data deficient” species make up around 17% of the nearly 150,000 species currently assessed.
When analysing conservation data study it is common for researchers to remove or underestimate assumptions of threat for these species, in order to control for unknown variations or misjudgements. Now, these researchers in Norway have tried to shed light on the black hole of unknown extinction risk by designing a machine learning model that predicts the threat of extinction for these data deficient species.
Machine learning for extinction assessment
When thinking of artificial intelligence and machine learning it is easy to imagine robots, computer-simulations and facial recognition. In reality, at least in ecological science, machine learning is simply an analytical tool used to run thousands of calculations to best represent the real-world study data we have.
In this case, the Norwegian researchers simplified the Red List extinction categories into a “binary classifier” model to predict a probability of whether data deficient species are likely “threatened” or “not threatened” by extinction. The model algorithm has “learned” from mathematical patterns found in biological and bioclimatic data of those species with an already assigned conservation category on the Red List.
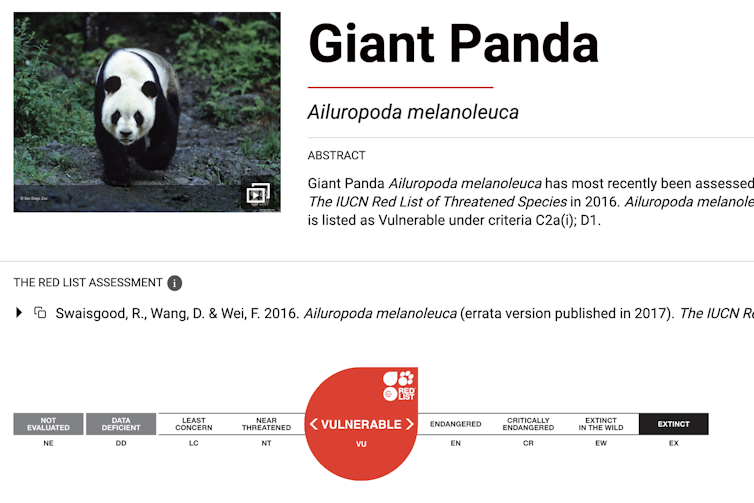
On land, these likely threatened terrestrial species are found across all continents, but live in small geographically restricted areas. This finding supports previous research with similar conclusions that species with small range sizes are particularly vulnerable to anthropogenic habitat degradation, such as deforestation or urbanisation.
At risk amphibians
Amphibians are the most at-risk group, with 85% of those data deficient species predicted as threatened (compared to 41% of those currently evaluated on the Red List). Amphibians are already a poster-child for the extinction crisis and are a key indicator for ecological health, as they depend on both land and water. We don’t know enough about what causes such catastrophic extinction of amphibians, and I am part of a science initiative trying to address the problem.
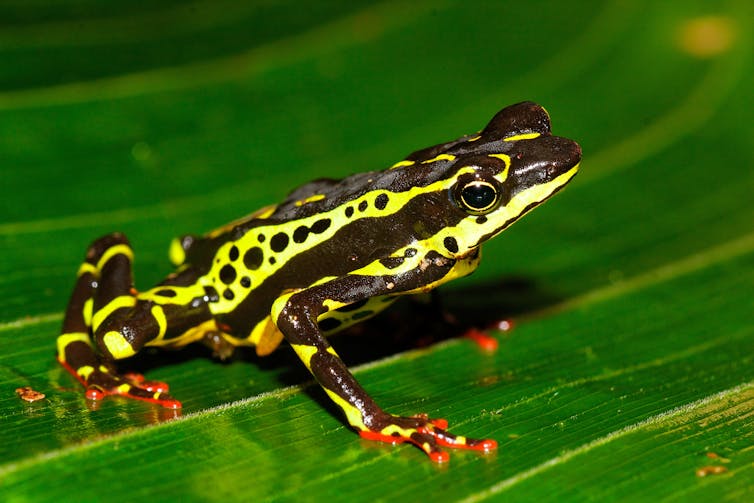
It’s a slightly different, but still tragic, story at sea. Data deficient marine species that are predicted to be facing extinction are concentrated along coasts, particularly in south-eastern Asia, the eastern Atlantic coastline and in the Mediterranean. When data deficient species are combined with fully-assessed species on the Red List, there is a 20% increase in the probability of extinction along the eastern coastlines of tropical Latin America.
What this means for global conservation
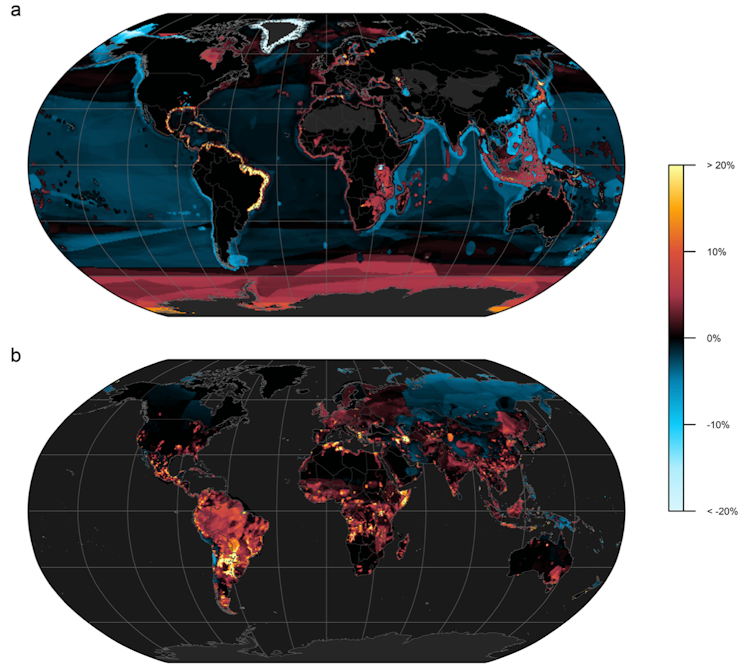
Though it is likely that the need for conservation has actually been underestimated worldwide these probability predictions are highly variable across different areas and groups of species, so don’t be fooled into over generalising these findings. But these broad results do highlight why it is so important to further investigate data deficient species.
The use of machine learning tools can be a time-and-cost-effective way to enhance the Red List and help overcome the challenging decision of where and what to protect, aiding targeted conservation action and expanding protected areas in these black holes of biodiversity.